The future of AI in education: 13 things we can do
Back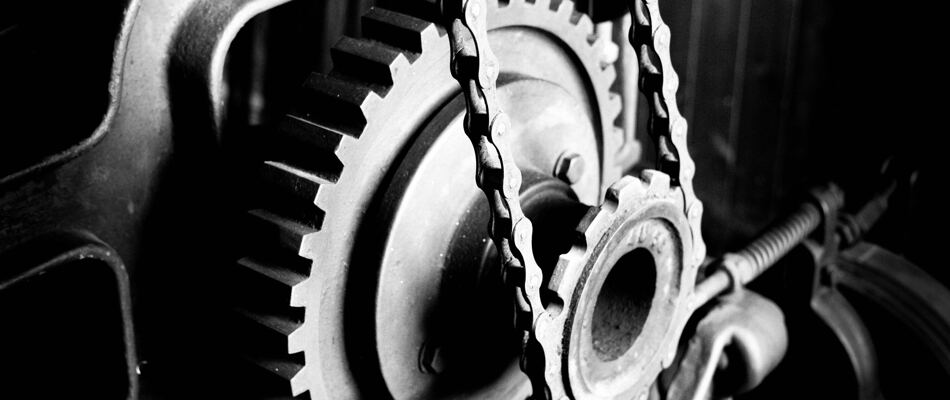
This piece was originally published as the introduction to a working paper entitled 'The future of Ai in education: 13 things we can do to minimise the damage' by Dr Arran Hamilton and professors John Hattie and Dylan Wiliam.
Throughout history, humans have fantasized about the possibility of creating thinking machines from inanimate mechanical parts. The ancient Greeks told mythical stories about Talos – a giant bronze automaton constructed by the god of blacksmiths. Leonardo De Vinci sketched drawings of humanoid robots; Isaac Asimov introduced a mechanical rogue villain in I Robot (1950); and in 1968 Arthur C. Clarke showed the take-over power of the artificially intelligent HAL in 2001: A Space Odessey, which was set 22 years in our past!
But all of this seemed like an utter fantasy, until 1956 when John McCarthy, Marvin Minsky, Nathaniel Rochester, and Claude Shannon came together for six weeks at the Dartmouth Conference to establish the field of Artificial Intelligence (AI). At the time, they were supremely optimistic that it would be possible to develop human-level thinking machines within a matter of years. Policymakers also took the prospect extremely seriously, with Lyndon B. Johnson establishing the US National Commission on Technology, Automation, and Economic Progress in 1964 (United States, 1966).
Yet despite these high aspirations, there was little progress until the late 1990s, and even after Deep Blue beat Gary Kasparov at chess in 1997, many remained sceptical that it would ever be possible to develop general artificial intelligence that would be able to think like us.
Within the last few years, however, opinions have changed, particularly with the arrival of Large Language Models. In November 2022, OpenAI launched ChatGPT-3 to the public, which did surprisingly well at many tasks that required higher-order thinking skills (and years of expensive education). ChatGPT-3 was quickly followed by GPT-3.5, and then by GPT-4 —each a noticeable improvement on its predecessor, and many other companies have developed their own Large Language Models.
In addition to the attention AI is now receiving in the mainstream media, it is also generating much interest in the world of education, teaching, and learning. Our concern, however, is that much of this is parochial. The focus is on issues in the here and now: tips and tricks to prompt the machines for better outputs; (reasonable) concerns about how to stop students using AI to cheat; and optimism that the technology can reduce teacher workload and act as a digital teaching aide for students. See Figure 1 for a summary of these near-view concerns.
Figure 1: AI Benefits and Concerns for Education
Benefits | Concerns |
Scaling quality education in hard to reach contexts; greater personalization; adaptive content; democratization of access; reductions in the cost of educational service delivery; teacher workload reductions; culturally relevant content; continuous assessment and feedback; coaching systems that help us to maintain goal commitment; decision support systems that help educational institutions to develop ‘good’ plans and stick to them; AI digital tutors instead of expensive high-intensity human tuition for learners with additional needs; AI support to teachers through augmented reality heads-up displays; deeper inferences about student learning through bio feedback tracking; faster identification and support for neuro-diverse learners; and multi- modal access for children with disabilities. | Accuracy (producing confident but incorrect answers); content bias; data protection, privacy and protection; plagiarism; surveillance of students and teachers; systems using poor pedagogic reasoning to increase pace of instruction for some students and to reduce it for others; equity of outcomes; algorithmic discrimination – where systems are used to make enrolment decisions/identify students for additional support; AI systems and tools that make decisions where it is impossible to understand how they are made; and worries that AI models take little account of context, and are focused on shallow factual knowledge. |
Source: author adaptation from US Department for Education Office of Educational Technology (2023); UNESCO (2021, 2022 & 2023); Giannini (2023).
These are all significant concerns, but we think there are much more serious issues that are receiving too little attention:
The future of education and learning!
Do schools and universities have a future, or will the machines soon be able to do everything we can – and better? Within the last decade, many educational policymakers were suggesting that we should teach students how to program, although there were others, such as Andreas Schleicher (the OECD’s head of education) arguing that it was a waste of time, as machines would be as good as humans at such tasks (Schleicher, 2019). But what if machines quickly advanced to a level where we could not even equal them or fully understand what they were doing in literally every domain? Would this new world leave us with a profound motivation gap and the risk we become permanently de-skilled and de-educated?
Thankfully the future is not (yet) set in stone. There are many different possibilities. Some have us still firmly in the driving seat, leveraging our education and collective learnings. However, in some of the other – less positive – possible futures, humanity might lose its critical reasoning skills, because the advice of the machines is always so good, so oracle-like, that it becomes pointless to think for ourselves, or to learn.
But to discuss these issues we think it is helpful to begin with a short explanation of how the Large Language Models (LLMs) that dominate current work on artificial intelligence—ChatGPT, Google Bard, Claude, and Meta LLaMA —work; what they are capable of; how they are similar/different to human brains; and what the implications might be for human learning and motivations to learn. This is the focus of Part One of the paper. In Part Two, we explore four different scenarios for humanity and in particular, what each of these scenarios might mean for the future of learning and education. Finally, in Part Three, we present 13 recommendations that we think will help to ensure that AI becomes our greatest success, rather than a tangled mess. Figure 2 provides a summary of the paper.
Figure 2: A Summary of the Paper
Waypoint | Key Point |
Part One: The Discombobulating Background | |
1.1 How do our brains work? | Our brains are largely about wiring and firing and this can be thought of as a kind of computational process. |
1.2 How do AI Large Language Models work? | These systems direct attention, plan, reason and regulate themselves in ways that are similar to the way our brains carry out these functions. |
1.3 But aren’t humans much more than machines? | Many of our distinctly human capabilities, such as emotion, empathy, and creativity can be explained and modelled by algorithms, so that machines can increasingly pretend to be like us. |
1.4 What is the current capability of these AI Systems? | These systems already exceed the ‘average’ human in a number of domains—perhaps a middling postgraduate but still prone to bouts of error. |
1.5 Have these AI systems reached their peak potential? | No, we should expect them to greatly surpass human reasoning capabilities – possibly very rapidly; think thousands of geniuses thinking millions of things at once. Eventually their computational capacity is likely to exceed the combined brainpower of every human that has ever lived. |
1.6 What has happened to human skills during other eras of technological innovation? | They were eroded, but this allowed us to focus on learning better/higher-order things. |
1.7 What are the Implications for human skills development the AI Era? | Experts may—initially at least—see their capabilities considerably amplified, but novices could become forever stunted. We might even be living in the era of ‘peak education’. As AI capabilities grow, our incentives to learn might diminish. It is not inconceivable that many of us might even lose the ability to read and write as these skills would, for many, serve no useful purpose in day-to-day living. |
Part TWO: Four Long-Term ScenariosThese scenarios speculate about potential futures for employment, education, and humanity – given what we have already unpacked in the discombobulating background of Part One. |
Scenario 1: AI is banned. | Governments come together and ban future developments in AI. --- However, if future developments in AI were banned humans would still be in the driving seat and still require education. There might also be significant benefits for human learning from leveraging the AI systems that have been developed so far and that might, subject to satisfactory guardrails, be excluded from any ban. |
Scenario 2: AI and Humans work side-by-side (a.k.a. Fake Work). | The AI gets so good that it can do most, if not all, human jobs. But governments legislate to force companies to keep humans in the labour market, to give us a reason to get up in the morning. We think this scenario is possible in the medium-term – beyond 2035 – as Large Language Models and other forms of AI become ever more sophisticated. But it may be highly dispiriting for humans to be ‘in the room’ whilst AI makes the decisions and no longer being at the forefront of ideas or decision-making. Even with increased education we would be unlikely to overcome this, or to think at the machines' speed and levels of sophistication. |
Scenario 3: Transhumanism where we upgrade our brains. | We choose to upgrade ourselves through brain-computer interfaces to compete with the machines and remain in the driving seat. There would also no longer be any need for schooling or university, because we could ‘download’ new skills from the cloud. |
Scenario 4: Universal Basic Income (UBI). | We decouple from the economy, leaving the machines to develop all the products and services; and to make all the big decisions. And we each received a monthly ‘freedom dividend’ to spend as we wish. --- It would enable us to save our ‘humanity’ by rejecting digital implants but potentially we would have no reason to keep learning, with most of the knowledge work and innovation being undertaken by the machines. We might pass the time playing parlour games, hosting grand balls, or learning and executing elaborate rituals. We would perhaps also become ever more interested in art, music, dance, drama, and sport. |
Where do we collectively go from here? 13 RecommendationsThese recommendations propose regulations to slow down the rate of AI advancement, so that we can collectively think and agree which of the four scenarios (or other destinations) suits humanity best. Otherwise, the decision will be taken for us, through happenstance and it may be almost impossible to reverse. We offer these as stimulus for further debate rather than as a final, definitive proposals but we believe that we need to conclude that debate FAST. | |
Again, there will be differences of opinion about some of these – so you can treat them more as stimulus to further debate, rather than as a final set of cast-iron proposals. But we need to have that debate FAST and then enact pragmatic measures that give us breathing room to decide what kind of future we want for humanity – before it is simply foisted upon us. |
Before we scare you too much, however, with our four scenarios – as noted above, we do not believe that the future is pre-determined. There are many other possible outcomes with different (happier) endings. However, we think we all need to understand the dystopian possibilities before we accidentally venture down a path with no escape route. This is more important than ever as we are arguably at what Will MacAskill (2022) calls ‘the hinge point of history’ i.e., that moment where things accelerate faster than ever before, where things move, for example, from linear to exponential. It is this that motivates our recommendations, helping to slow down the rate of progress and collectively giving us time to think.
Some bullish researchers already think we may only be two years out from Artificial General Intelligence that can reason to the same standard as you or us (Cotra, 2023). Most others – the bearish – still think it will likely be with us before 2040 i.e., the time at which today’s toddlers graduate from high-school; and quite possibly sooner (Roser, 2023).
Our own position is that there is great uncertainty but that we ALL need to maintain a stance of vigilance and assume – from now on – that at any moment in time we could be only two years out from machines that are at least as capable as us. So, we can’t bury our heads in the sand or get all parochial – we need to grapple with these ideas and their implications today.
References
Cotra, A. (2023). Two-year update on my personal AI timelines. AI Alignment Forum. Two-year update on my personal AI timelines — AI Alignment Forum
Giannini, S. (2023). Reflections on generative AI and the future of education. UNESCO. Generative AI and the future of education - UNESCO Digital Library
Roser, M. (2023). AI timelines: What do experts in artificial intelligence expect for the future? Our World in Data. AI timelines: What do experts in artificial intelligence expect for the future? - Our World in Data
Schleicher, A. (2019). Should schools teach coding? OECD Education and Skills Today, Should schools teach coding? - OECD Education and Skills Today (oecdedutoday.com)
U.S. Department of Education, Office of Educational Technology. (2023) Artificial Intelligence and Future of Teaching and Learning: Insights and Recommendations, Washington, https://tech.ed.gov/
UNESCO. (2021). AI and education Guidance for policy-makers. UNESCO.
UNESCO. (2022). Recommendation on the Ethics of Artificial Intelligence. UNESCO.
UNESCO. (2023). ChatGPT and Artificial Intelligence in Higher Education Quick start guide. UNESCO.
United States. (1966). National Commission on Technology, Automation, and Economic Progress. Automation and Economic Progress. U.S. Government Printing Office.